Is There Racial Bias in Twitter’s Image Previews?
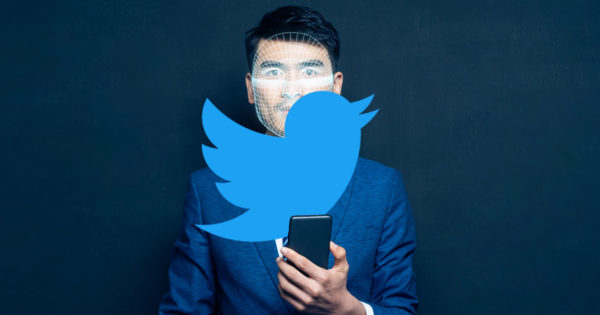
Over the weekend, Twitter users began to notice something potentially prejudiced about the way the social network’s algorithm crops photos for thumbnails: Post an image containing two headshots—one of a person of color and one of a white person—and the automatic feature seems more likely to zoom in on the latter in the image preview.
The informal round of product testing began with a now-viral post in which tech worker Tony Arcieri demonstrated how Twitter repeatedly zeroed in on Sen. Mitch McConnell’s face in images containing both his and former President Barack Obama’s headshots.
Twitter sleuths quickly began to run their own experiments to repeat the results for themselves or to test for other types of bias. Some even found that the algorithm was seemingly more likely to choose white animals over black animals.
Twitter has since said that it would revisit the algorithm in an open-source format following the outcry. A spokesperson said the model cleared tests for racial and gender bias before it was first rolled out in early 2018, but that “it’s clear that we’ve got more analysis to do.”
“This is a very important question,” Twitter chief technology officer Parag Agrawal said in a separate tweet. “To address it, we did analysis on our model when we shipped it, but needs continuous improvement. Love this public, open, and rigorous test — and eager to learn from this.”
Twitter switched its image thumbnail system from a simple face detection tool to an AI trained to identify “salient” areas of a given image—pixels more likely to naturally attract the human eye as determined by eye trackers—in early 2018, according to a blog post detailing the change.
“This lets us perform saliency detection on all images as soon as they are uploaded and crop them in real time,” Twitter machine learning engineers wrote in the blog post.
The nature of black-box AI algorithms makes it difficult to discern the factors in any particular decision a neural network makes without extensive testing, which is why experts on AI bias often emphasize the need for thorough vetting at every stage of development. In this case, the perceived bias could be rooted in anything from how the human eye perceives color to racial biases documented in various academic studies on eye gaze tracking.
Anima Anandkumar, director of AI research for Nvidia and Caltech machine learning professor, said the difficulty to trace a machine’s reasoning and check for any possible scenario makes the vetting process difficult.
“This is a universal problem with testing AI,” Anandkumar said. “There are so many edge cases (long tail) in the real world. Current deep learning methods make it impossible to easily discover those during testing because of its black-box nature. There is both bias in data and DL methods tend to amplify and obfuscate the problem.”
Anandkumar said a key question is determining who the test subjects for the training were. “If they are predominantly straight white men who are ogling at women’s chest and preferring to look at white skin, we have a huge problem that their gaze becomes universal,” Anandkumar said. “We are now all co-opting their gaze at a universal scale.”
The conversation comes as discussion over how AI can reflect the human biases of its input data or the developers who programmed it has gained traction, particularly in the realm of image recognition. In June, a machine learning tool designed to de-pixelate images was found to have an unfortunate tendency to whitewash people of color in its output, kicking off a wide-ranging debate among AI researchers over the possible causes of such bias.
https://www.adweek.com/digital/whats-going-on-seemingly-racially-biased-twitter-previews/