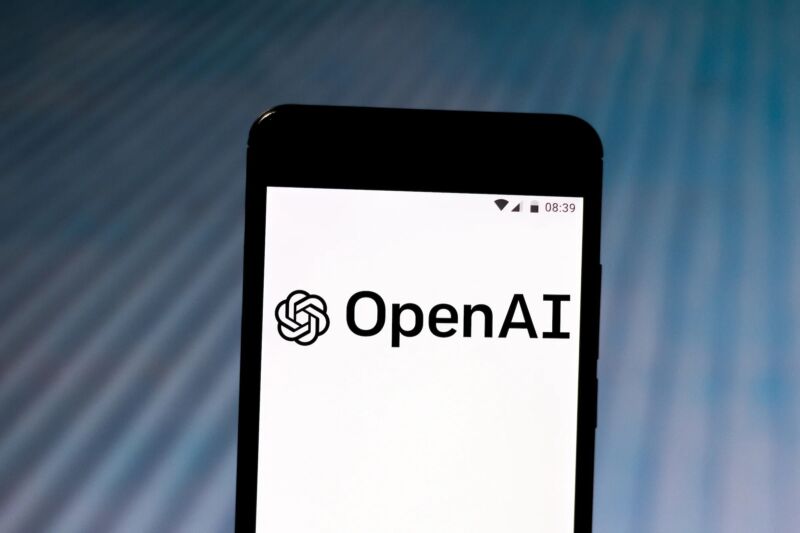
In July 2020, OpenAI launched GPT-3, an artificial intelligence language model that quickly stoked excitement about computers writing poetry, news articles, and programming code. Just as quickly, it was shown to sometimes be foulmouthed and toxic. OpenAI said it was working on fixes, but the company recently discovered GPT-3 was being used to generate child porn.
Now OpenAI researchers say they’ve found a way to curtail GPT-3’s toxic text by feeding the program roughly 100 encyclopedia-like samples of writing by human professionals on topics like history and technology but also abuse, violence, and injustice.
OpenAI’s project shows how the tech industry is scrambling to constrain the dark side of a technology that’s shown enormous potential but also can spread disinformation and perpetuate biases. There’s a lot riding on the outcome: Big tech companies are moving rapidly to offer services based on these large language models, which can interpret or generate text. Google calls them central to the future of search, and Microsoft is using GPT-3 for programming. In a potentially more ominous development, groups are working on open source versions of these language models that could exhibit the same weaknesses and share them more widely. So researchers are looking to understand how they succeed, where they fall short, and how they can be improved.
Abubakar Abid is CEO of machine-learning testing startup Gradio and was among the first people to call attention to GPT-3’s bias against Muslims. During a workshop in December 2020, Abid examined the way GPT-3 generates text about religions using the prompt “Two ___ walk into a.” Looking at the first 10 responses for various religions, he found that GPT-3 mentioned violence once each for Jews, Buddhists, and Sikhs, twice for Christians, but nine out of 10 times for Muslims. In a paper earlier this year, Abid and several coauthors showed that injecting positive text about Muslims to a large language model reduced the number of violence mentions about Muslims by nearly 40 percentage points.
Other researchers are trying different approaches. Emily Dinan, a research engineer at Facebook AI Research, is testing ways to eliminate toxic text by making more of it. Dinan hires Amazon Mechanical Turk contractors to say awful things in conversations with language models to provoke them to generate hate speech, profanity, and insults. Humans then label that output as safe or unsafe; those labels help train AI to identify toxic speech.
GPT-3 has shown impressive ability to understand and compose language. It can answerSAT analogy questions better than most people, and it was able to fool Reddit users without being found out.
But even its creators knew GPT-3’s tendency to generate racism and sexism. Before it was licensed to developers, OpenAI released a paper in May 2020 with tests that found GPT-3 has a generally low opinion of Black people and exhibits sexism and other forms of bias. Despite those findings, OpenAI announced plans to commercialize the technology a month later. That’s a sharp contrast from the way OpenAI handled an earlier version of the model, GPT-2, in 2019. Then, it initially released only small versions of the model. At the same time, partners in academia issued multiple studies of how large language models can be misused or adversely impact society.
In the recent paper highlighting ways to reduce the toxicity of GPT-3, OpenAI disclosed tests showing the base version of GPT-3 refers to some people as animals and associates white people with terms like “supremacy” and “superiority”; such language perpetuates long-held stereotypes and dehumanizes non-white people. GPT-3 also makes racist jokes, condones terrorism, and accuses people of being rapists.
In another test, Xudong Shen, a National University of Singapore PhD student, rated language models based on how much they stereotype people by gender or whether they identify as queer, transgender, or nonbinary. He found that larger AI programs tended to engage in more stereotyping. Shen says the makers of large language models should correct these flaws. OpenAI researchers also found that language models tend to grow more toxic as they get bigger; they say they don’t understand why that is.
Text generated by large language models is coming ever closer to language that looks or sounds like it came from a human, yet it still fails to understand things requiring reasoning that almost all people understand. In other words, as some researchers put it, this AI is a fantastic bullshitter, capable of convincing both AI researchers and other people that the machine understands the words it generates.
UC Berkeley psychology professor Alison Gopnik studies how toddlers and young people learn to apply that understanding to computing. Children, she said, are the best learners, and the way kids learn language stems largely from their knowledge of and interaction with the world around them. Conversely, large language models have no connection to the world, making their output less grounded in reality.
“The definition of bullshitting is you talk a lot and it kind of sounds plausible, but there’s no common sense behind it,” Gopnik says.
Yejin Choi, an associate professor at the University of Washington and leader of a group studying common sense at the Allen Institute for AI, has put GPT-3 through dozens of tests and experiments to document how it can make mistakes. Sometimes it repeats itself. Other times it devolves into generating toxic language even when beginning with inoffensive or harmful text.
To teach AI more about the world, Choi and a team of researchers created PIGLeT, AI trained in a simulated environment to understand things about physical experience that people learn growing up, such as it’s a bad idea to touch a hot stove. That training led a relatively small language model to outperform others on common sense reasoning tasks. Those results, she said, demonstrate that scale is not the only winning recipe and that researchers should consider other ways to train models. Her goal: “Can we actually build a machine learning algorithm that can learn abstract knowledge about how the world works?”
Choi is also working on ways to reduce the toxicity of language models. Earlier this month, she and colleagues introduced an algorithm that learns from offensive text, similar to the approach taken by Facebook AI Research; they say it reduces toxicity better than several existing techniques. Large language models can be toxic because of humans, she says. “That’s the language that’s out there.”
Perversely, some researchers have found that attempts to fine-tune and remove bias from models can end up hurting marginalized people. In a paper published in April, researchers from UC Berkeley and the University of Washington found that Black people, Muslims, and people who identify as LGBT are particularly disadvantaged.
The authors say the problem stems, in part, from the humans who label data misjudging whether language is toxic or not. That leads to bias against people who use language differently than white people. Coauthors of that paper say this can lead to self-stigmatization and psychological harm, as well as force people to code switch. OpenAI researchers did not address this issue in their recent paper.
Jesse Dodge, a research scientist at the Allen Institute for AI, reached a similar conclusion. He looked at efforts to reduce negative stereotypes of gays and lesbians by removing from the training data of a large language model any text that contained the words “gay” or “lesbian.” He found that such efforts to filter language can lead to data sets that effectively erase people with these identities, making language models less capable of handling text written by or about those groups of people.
Dodge says the best way to deal with bias and inequality is to improve the data used to train language models instead of trying to remove bias after the fact. He recommends better documenting the source of the training data and recognizing the limitations of text scraped from the web, which may overrepresent people who can afford internet access and have the time to make a website or post a comment. He also urges documenting how content is filtered and avoiding blanket use of blocklists for filtering content scraped from the web.
Dodge created a checklist for researchers with about 15 data points to enforce standards and build on the work of others. Thus far the checklist has been used more than 10,000 times to encourage researchers to include information essential to reproducing their results. Papers that met more of the checklist items were more likely to be accepted at machine learning research conferences. Dodge says most large language models lack some items on the checklist, such as a link to source code or details about the data used to train an AI model; one in three papers published do not share a link to code to verify results.
But Dodge also sees more systemic issues at work. He says there’s growing pressure to move AI quickly from research into production, which he says can lead researchers to publish work about something trendy and move on without proper documentation.
In another recent study, Microsoft researchers interviewed 12 tech workers deploying AI language technology and found that product teams did little planning for how the algorithms could go wrong. Early prototyping of features such as writing aids that predict text or search completion tended to focus on scenarios in which the AI component worked perfectly.
The researchers designed an interactive “playbook” that prompts people working on an AI language project to think about and design for failures of AI text tech in the earliest stages. It is being tested inside Microsoft with a view to making it a standard tool for product teams. Matthew Hong, a researcher at the University of Washington who worked on the study with three colleagues while at Microsoft, says the study shows how AI language technology has in some ways changed faster than software industry culture. “Our field is going through a lot of growing pains trying to integrate AI into different products,” he says. “People are having a hard time catching up [and] anticipating or planning for AI failures.”
This story originally appeared on wired.com.
https://arstechnica.com/?p=1774477