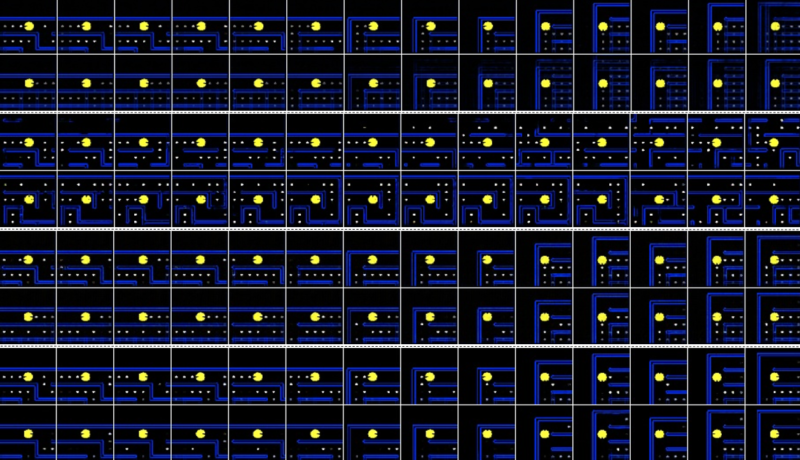
You may be familiar with the Infinite Monkey Theorem, an oft-cited (and often incorrectly quoted) claim that thousands of monkeys could bang on thousands of typewriters and eventually produce a work of art equivalent to William Shakespeare. (Yes, Simpsons did it.)
This week, Nvidia confirms that it has taken this theory quite seriously with its own twist: an army of AI routines, dubbed GameGAN (short for “generative adversarial networks”), trained to build a playable video game from scratch. More precisely, they’ve chosen one of the industry’s biggest, most recognizable games, celebrating its 40th anniversary today: Pac-Man.
If you’ve seen other farms of computers trained on existing games, this has usually come in the form of them learning how to play the game in question. After watching thousands of hours of a particular game and tracking the most successful moves and reactions in the course of a versus match, these AI routines can then control games, repeat and juggle thousands of strategies, and battle humans. (Sometimes the results go well for the computers, but not always.)
Nvidia’s latest experiment starts in similar fashion, as its AI research team trained a farm of four computers—each equipped with a Quadro GV100 workstation-grade GPU—on 50,000 hours of Pac-Man gameplay. That gameplay, conveniently enough, was also played by a separate AI. Trained on this footage, the computers in question then turned around and created their own identical-looking clone… with some exceptions.
Watch Pac-Man, write Pac-Man
“Our AI didn’t see any of [Pac-Man‘s] code, just pixels coming out of the game engine,” Nvidia representative Hector Marinez said to Ars Technica. “By watching this, it learned the rules.” Those included specifics like: Pac-Man’s speed, movement abilities, and inability to go through walls; the four ghosts’ movement patterns; what happens when Pac-Man eats a power pellet; and what happens when ghosts touch Pac-Man, super-charged or otherwise.
“Any of us could watch hours of people playing Pac-Man, and from that, you could potentially write your own Pac-Man game, just by observing the rules,” Marinez said. “That’s what this AI has done.”
Marinez and the rest of Nvidia’s available researchers did not clarify exactly what coding subroutine drove this AI’s ability to write executable code of its own, nor whether it leaned on existing game engines. (After all, if simply watching hours of Pac-Man could teach me how to code video games by hand, I would have a very different career.) Eventually, they handed us a copy of the associated paper that explains how Nvidia’s research team pulled it off. It’s… complicated. The quickest description is a series of three modules (memory, dynamics engine, and rendering engine) that run as neural networks.
The available researchers also admitted that Nvidia’s existing model suffers from fidelity issues. The below gameplay maxes out at roughly 128×128 pixel resolution, which runs at roughly 50 frames per second, below the series’ 60fps standard.
Nvidia says this playable version of Pac-Man will be made available to the public “this summer,” though the company would not clarify whether it would be distributed as a downloadable executable or served via a restricted cloud-gaming service like Nvidia’s GeForce Now.
In our interview, Nvidia suggested how this technology might some day revolutionize the work pipeline of a video game studio. The representatives didn’t do much to back up their bold claim that “there’s a straight line from Pac-Man to having GameGAN produce games and simulations” of the same quality as modern 3D mega-hits. But the team’s researchers were bullish on AI-driven routines possibly aiding the development of massive virtual worlds as tools to be directed by human staffers.
“We’ve created an AI agent that can learn the rules of the game just by observing it,” one Nvidia representative said. “Before you create a tool that puts content into the game, it needs to understand the rules.” They went on to discuss the wealth of assets (characters, buildings, vehicles) you might expect in a massive open-world game—which need to first be designed and then coded to react realistically to the game world around them. “Think of Grand Theft Auto V. Thousands of artists worked over many years to produce those worlds. Any tools to assist in generating that content would be very valuable.”
Full pretzel fidelity, some day?
But Nvidia didn’t offer a guess or estimate of how much a farm of computers would have to scale up to study and examine real-time gameplay of 3D games, let alone the kinds of videotaped, real-world environment study that the company could use to build smarter, more responsive real-world robots. It needed 50,000 hours to interpret a single Pac-Man maze, and again, that was choked up in terms of fidelity; one researcher admitted that higher resolutions are “still an open challenge for these kinds of networks.” How many equivalent processing hours will be needed to recreate a dated 3D game like Grand Theft Auto: San Andreas, let alone best-in-class 3D worlds?
We’re not the kinds to say it can’t be done. But while the cutting-edge idea has us intrigued, we’re going to reserve our excitement until we see a full-resolution version of Q-Bert get its own GameGAN translation. (Really, even the four-puzzle upgrade of Ms. Pac-Man would be a good start. Maybe in time for its 40th anniversary in 2022?)
https://arstechnica.com/?p=1678052