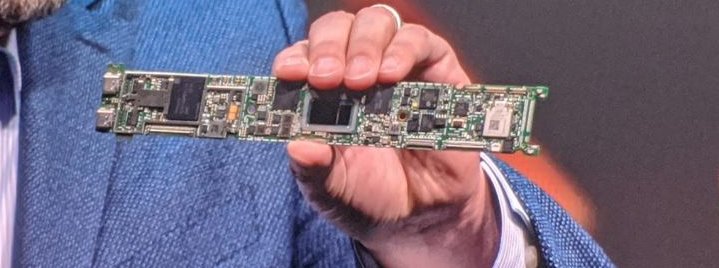
Yesterday at CES 2020, Intel previewed its next-generation line of mobile CPUs, code-named Tiger Lake, in several new form factors while running brand-new (and impressive) software designed with the platform in mind.
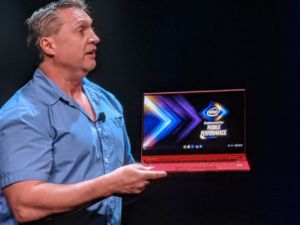
Tiger Lake plays into Intel’s ongoing Project Athena program, which aims to bring a performance and usability standard with concrete, testable metrics to mobile computing—that includes at least nine hours of battery life with the screen at 250 nits of brightness, out-of-the-box display and system settings, and multiple tabs and applications running. Project Athena has now been expanded to cover some new Chromebook models, as well as traditional Windows PCs.
Several new foldable designs were announced during the presentation, ranging from a relatively conventional Dell hinged two-in-one to much more outré designs such as Lenovo’s X1 Fold—presented onstage by Lenovo President Christian Teismann—and an Intel concept design prototype called Horseshoe Bend. Both the X1 Fold and Horseshoe Bend will look immediately familiar to anyone who has been following Ron Amadeo’s coverage of the Samsung and Motorola foldable smartphones; in each design, the screen itself folds down the middle.
-
This Dell two-in-one was the most conventional design shown last night: it uses discrete physical hinges, not a foldable screen.
-
This Dell two-in-one has a removable chiclet keyboard covering the bottom screen in this shot.Jim Salter
-
When unfolded, the Lenovo X1 Fold looks like a monstrous 17-inch tablet.Jim Salter
-
This action shot shows a Lenovo X1 Fold partially folded, with the bottom half of the screen acting as a full-size onscreen keyboard.Jim Salter
-
This half-folded Lenovo X1 Fold gives us a peek at an incredibly skeuomorphic “ebook reader.”Jim Salter
-
Unfolded, the Horseshoe Bend concept prototype looks like an enormous tablet. The screen crease isn’t extreme, but it is often visible.Jim Salter
-
Horseshoe Bend half-folded looks, for the most part, like a bigger version of the Lenovo X1 Fold.Jim Salter
The software demonstrations, presented by Adobe “Principal Worldwide Evangelist” Jason Levine, were by far the most compelling part of Intel’s mobile presentation. Levine performed three separate demonstrations of AI-empowered work using Adobe Sensei, with all of the manic energy of Vince Offer selling you a Slap Chop. Levine’s antics aside, the demonstrations were impressive—an automatic boundary selection of a bird in the foreground of a complex photo, another of a rose with significant light bloom muddying up its edges, and finally an automatic video conversion from landscape to portrait for a short clip of an extreme skier. The automatic selection of the bird and rose weren’t instant, but they took place in about five seconds apiece—far less time than it would take even the most skilled human artist to manually trace the edges of the photos—and appeared extremely high quality. Levine placed the cropped-out images into other scenes quickly after selecting them, with excellent visual results.
He also showed the audience a clip of an extreme skier, slaloming back and forth and doing flips across the entire field of a landscape video. Commenting about how many social media platforms were designed for portrait images, he then engaged an automatic conversion on Adobe Sensei, which automatically recognized the skier as “foreground” of the clip and panned the portrait frame automatically back and forth as necessary to keep the skier in center-frame on conversion.
-
Levine spends some time selling the crowd on how difficult it is to find the right boundaries to crop an image with hair or feathers.Jim Salter
-
The crowd gasped as Levine cropped out the background around the automatically boundary-selected bird, feathers and all.Jim Salter
-
Levine prepares to automatically select boundaries on a rose in the foreground of a photo, despite light rays muddying up the edges.Jim Salter
-
Jason effortlessly transports the cropped-out rose bloom into a new photo, scales it into place, and vamps for the crowd.Jim Salter
-
Levine demonstrates telling Adobe Sensei to automatically reformat a video from landscape to portrait mode, and “follow the foreground” in the cropped-down video.Jim Salter
-
After automatic conversion and reframing, the now-portrait video follows the skier back and forth perfectly.Jim Salter
After the entire set of demos was complete, we got the reveal that Levine had been performing them live using a Tiger-Lake equipped 13-inch ultralight notebook. All of the automatic selection, cropping, and panning work shown uses Intel’s OpenVINO AI framework and is greatly accelerated by its DLB x86 instruction-set extensions—so while the same tasks should run on non-Intel (and/or non-DLB-capable) hardware also, they’ll likely run several times slower.
When Ars tested the impact of Deep Learning Boost hands-on, benchmarking the i9-10980XE versus AMD’s much more powerful Threadripper 3970x, we saw the DLB-equipped i9-10980XE perform image-classification tasks at roughly double to quadruple the rate of either the Threadripper or Intel’s own non-DLB-equipped i9-9980XE. As AI-powered tasks become more and more common in applications ranging from Office to Photoshop, we expect that the ability to make short work of inference workloads will become nearly as important as general CPU performance itself.
https://arstechnica.com/?p=1640189