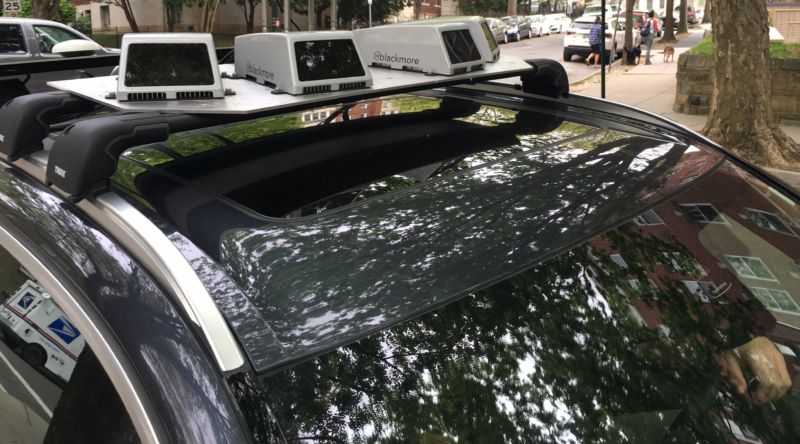
Fresh from a $530 million fundraising round earlier this year, self-driving startup Aurora made a big bet on lidar last week. The company—founded by veterans of Tesla and of Google’s self-driving car projects—scooped up a Montana-based lidar startup called Blackmore.
Lidar sensors have a lot in common with fiber-optic communications gear. Both work by sending out information encoded in light, then recapturing it and interpreting the information it contains.
Blackmore’s leaders have deep ties to the optical telecom industry, and the company aims to pack more and more components of its lidar sensors into photonic integrated circuits that have been pioneered in the optical telecom sector. These circuits are expensive to design but affordable to manufacture at scale. Earlier this year, Blackmore’s most powerful lidar sensors cost as much as $20,000. But by the time Aurora is ready to start selling its self-driving stack to automakers, Blackmore lidar is slated to cost a fraction of that price.
Another big selling point for Blackmore is its approach to measuring distances—called frequency-modulated continuous wave (FMCW).
FMCW is Blackmore’s secret weapon
Conventional lidar systems measure distances by sending out a short laser pulse and then measuring how long it takes for the beam to bounce back. Blackmore’s FMCW lidar uses a more complex method.
A FMCW lidar works by steadily changing the frequency of outgoing laser light. The outgoing beam is split into two parts, with one part traveling out into the world. When that beam bounces back, it is recombined with the other half.
At this point, the two beams have traveled different distances. And because the light frequency was changing, the two beams have different frequencies. Combining two beams with similar frequencies produces a beat frequency that depends on the frequency difference between the two beams. And if the beam frequency is changing in a predictable way, it’s straightforward to convert this frequency difference into a distance measurement.
This might seem like an unnecessarily complicated way to measure how a laser beam travels, but it has some significant advantages. One is that FMCW lidar tends to be more robust to interference from other light sources. Conventional time-of-flight lidar can become confused by glare from the sun or other noisy light sources. FMCW is a nimble approach that’s less likely to get confused by external light sources.
Blackmore also argues that the FMCW approach is a perfect fit for lidar based on optical telecom components. Traditional time-of-flight lidar depends on the ability to produce short, powerful laser bursts—bursts that could burn out delicate components in a photonic integrated circuit. As the name frequency modulated continuous wave suggests, FMCW lidar doesn’t tend to generate big power spikes.
FMCW lidar measures both distance and velocity
Perhaps the most important advantage of FMCW lidar is its ability to measure the velocities of nearby objects and their distances simultaneously.
When light bounces off a moving object, its frequency is increased or decreased by a small amount—a phenomenon known as a Doppler shift. This complicates distance measurements, since there’s no way for a single measurement to distinguish a frequency change caused by longer distance from a change caused by an object in motion.
FMCW lidar deals with this by taking multiple measurements—for example, a measurement with a rising frequency followed by one with a falling frequency. This switches the sign of the relationship between distance and frequency between the two measurements, while the relationship between velocity and frequency remains unchanged. Then it just takes a bit of algebra to determine both the velocity and the distance of a particular object. (In practice, FMCW lidars tend to vary their frequencies in more complex ways, the details of which are closely held secrets.)
I got to see this capability in action last month when a couple of Blackmore staffers stopped by my neighborhood in Washington, DC. I rode in an SUV with four Blackmore lidar units on the roof and was able to look at a display showing the lidar’s output in real time.
Like any lidar, the screen displayed a three-dimensional “point cloud” showing the location of objects near the vehicle. But this point cloud had something unique: each point was color-coded to indicate its velocity toward or away from the lidar sensor.
This color-coding meant that I could distinguish moving objects from stationary ones at a glance. Cars, bicycles, and other moving objects on the road showed as a different color than points that represented parked cars, trees, the road, and other stationary objects.
This ability seems particularly valuable at long distances. At a range of 200 meters, a car might be represented by only a handful of pixels—too few to identify it as a car based on its shape. But velocity measurements make it easy to distinguish moving objects from stationary ones. This is particularly crucial at freeway speeds since a car can close a distance of 200 meters in just a few seconds.
And the display’s velocity-based color coding provided other clues about what kinds of objects I was looking at. For example, the lidar was sensitive enough to detect that the top and bottom of a car’s wheels were moving at different speeds than the rest of the vehicle. The top of the wheel moved faster than the rest of the car, while the bottom moved relatively slowly.
Pedestrians exhibited their own unique patterns of varied velocities, with their arms and legs alternatively moving faster and slower than their torsos.
Hence, accurate velocity measurements based on Doppler shifts provide a type of data that no other kind of sensor can match. Automotive radar has long made velocity measurements using similar techniques, but these radars have much coarser angular resolution. Doppler radar can tell that there’s something moving 20 miles per hour ahead, but it can tell very little about what kind of object it is or exactly where it’s located. Conventional lidar can provide more accurate measurements of a car’s location, but it can make only crude velocity measurements by comparing successive distance measurements.
Blackmore isn’t the only startup developing FMCW lidar, but it’s a fairly small club. The company’s best-known rival in the FMCW lidar market is Aeva, founded by a couple of veterans of Apple’s still-secret autonomy project. Strobe, a lidar startup acquired by GM in 2017, may also be working on FMCW lidar, though the company hasn’t revealed many details about its technology.
https://arstechnica.com/?p=1511485