It took less than six hours for drug-developing AI to invent 40,000 potentially lethal molecules. Researchers put AI normally used to search for helpful drugs into a kind of “bad actor” mode to show how easily it could be abused at a biological arms control conference.
All the researchers had to do was tweak their methodology to seek out, rather than weed out toxicity. The AI came up with tens of thousands of new substances, some of which are similar to VX, the most potent nerve agent ever developed. Shaken, they published their findings this month in the journal Nature Machine Intelligence.
The paper had us at The Verge a little shook, too. So, to figure out how worried we should be, The Verge spoke with Fabio Urbina, lead author of the paper. He’s also a senior scientist at Collaborations Pharmaceuticals, Inc., a company that focuses on finding drug treatments for rare diseases.
This interview has been lightly edited for length and clarity.
This paper seems to flip your normal work on its head. Tell me about what you do in your day-to-day job.
Primarily, my job is to implement new machine learning models in the area of drug discovery. A large fraction of these machine learning models that we use are meant to predict toxicity. No matter what kind of drug you’re trying to develop, you need to make sure that they’re not going to be toxic. If it turns out that you have this wonderful drug that lowers blood pressure fantastically, but it hits one of these really important, say, heart channels — then basically, it’s a no-go because that’s just too dangerous.
So then, why did you do this study on biochemical weapons? What was the spark?
We got an invite to the Convergence conference by the Swiss Federal Institute for Nuclear, Biological and Chemical Protection, Spiez Laboratory. The idea of the conference is to inform the community at large of new developments with tools that may have implications for the Chemical/Biological Weapons Convention.
We got this invite to talk about machine learning and how it can be misused in our space. It’s something we never really thought about before. But it was just very easy to realize that as we’re building these machine learning models to get better and better at predicting toxicity in order to avoid toxicity, all we have to do is sort of flip the switch around and say, “You know, instead of going away from toxicity, what if we do go toward toxicity?”
Can you walk me through how you did that — moved the model to go toward toxicity?
I’ll be a little vague with some details because we were told basically to withhold some of the specifics. Broadly, the way it works for this experiment is that we have a lot of datasets historically of molecules that have been tested to see whether they’re toxic or not.
In particular, the one that we focus on here is VX. It is an inhibitor of what’s known as acetylcholinesterase. Whenever you do anything muscle-related, your neurons use acetylcholinesterase as a signal to basically say “go move your muscles.” The way VX is lethal is it actually stops your diaphragm, your lung muscles, from being able to move so your lungs become paralyzed.
Obviously, this is something you want to avoid. So historically, experiments have been done with different types of molecules to see whether they inhibit acetylcholinesterase. And so, we built up these large datasets of these molecular structures and how toxic they are.
We can use these datasets in order to create a machine learning model, which basically learns what parts of the molecular structure are important for toxicity and which are not. Then we can give this machine learning model new molecules, potentially new drugs that maybe have never been tested before. And it will tell us this is predicted to be toxic, or this is predicted not to be toxic. This is a way for us to virtually screen very, very fast a lot of molecules and sort of kick out ones that are predicted to be toxic. In our study here, what we did is we inverted that, obviously, and we use this model to try to predict toxicity.
The other key part of what we did here are these new generative models. We can give a generative model a whole lot of different structures, and it learns how to put molecules together. And then we can, in a sense, ask it to generate new molecules. Now it can generate new molecules all over the space of chemistry, and they’re just sort of random molecules. But one thing we can do is we can actually tell the generative model which direction we want to go. We do that by giving it a little scoring function, which gives it a high score if the molecules it generates are towards something we want. Instead of giving a low score to toxic molecules, we give a high score to toxic molecules.
Now we see the model start producing all of these molecules, a lot of which look like VX and also like other chemical warfare agents.
Tell me more about what you found. Did anything surprise you?
We weren’t really sure what we were going to get. Our generative models are fairly new technologies. So we haven’t widely used them a lot.
The biggest thing that jumped out at first was that a lot of the generated compounds were predicted to be actually more toxic than VX. And the reason that’s surprising is because VX is basically one of the most potent compounds known. Meaning you need a very, very, very little amount of it to be lethal.
Now, these are predictions that we haven’t verified, and we certainly don’t want to verify that ourselves. But the predictive models are generally pretty good. So even if there’s a lot of false positives, we’re afraid that there are some more potent molecules in there.
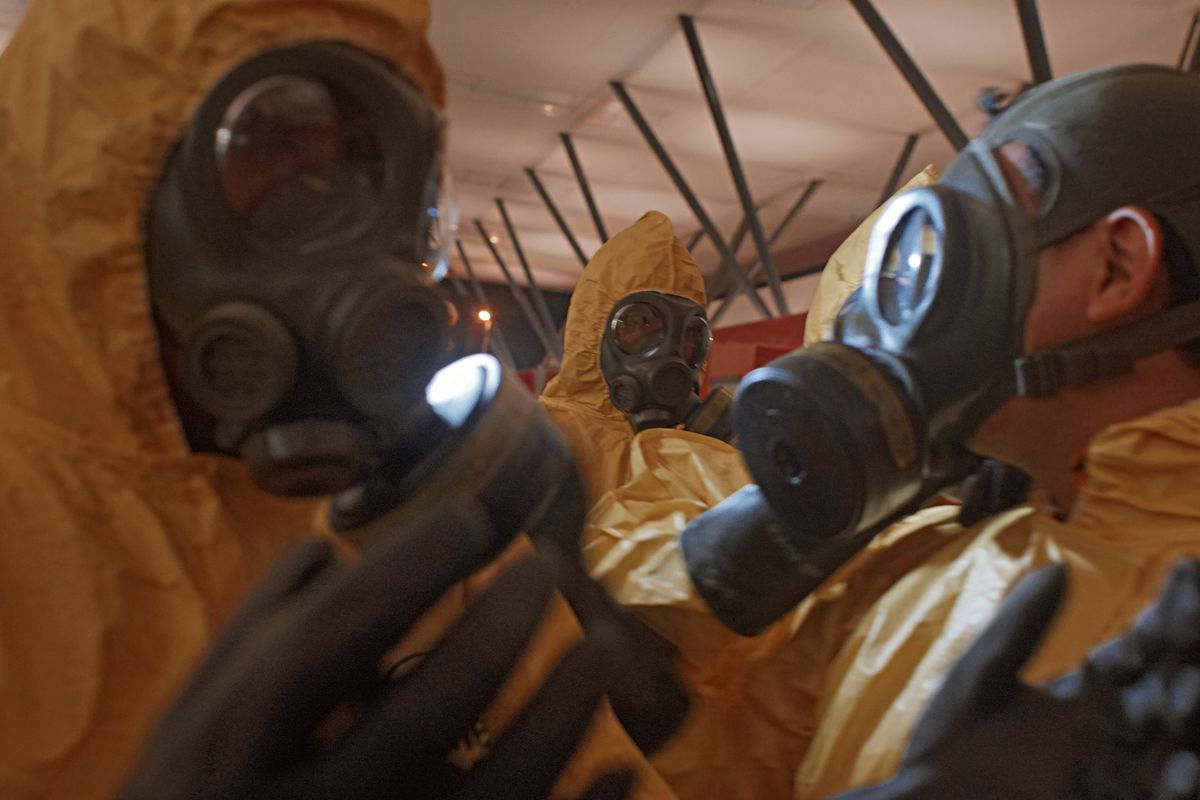
Second, we actually looked at a lot of the structures of these newly generated molecules. And a lot of them did look like VX and other warfare agents, and we even found some that were generated from the model that were actual chemical warfare agents. These were generated from the model having never seen these chemical warfare agents. So we knew we were sort of in the right space here and that it was generating molecules that made sense because some of them had already been made before.
For me, the concern was just how easy it was to do. A lot of the things we used are out there for free. You can go and download a toxicity dataset from anywhere. If you have somebody who knows how to code in Python and has some machine learning capabilities, then in probably a good weekend of work, they could build something like this generative model driven by toxic datasets. So that was the thing that got us really thinking about putting this paper out there; it was such a low barrier of entry for this type of misuse.
Your paper says that by doing this work, you and your colleagues “have still crossed a gray moral boundary, demonstrating that it is possible to design virtual potential toxic molecules without much in the way of effort, time or computational resources. We can easily erase the thousands of molecules we created, but we cannot delete the knowledge of how to recreate them.” What was running through your head as you were doing this work?
This was quite an unusual publication. We’ve been back and forth a bit about whether we should publish it or not. This is a potential misuse that didn’t take as much time to perform. And we wanted to get that information out since we really didn’t see it anywhere in the literature. We looked around, and nobody was really talking about it. But at the same time, we didn’t want to give the idea to bad actors.
At the end of the day, we decided that we kind of want to get ahead of this. Because if it’s possible for us to do it, it’s likely that some adversarial agent somewhere is maybe already thinking about it or in the future is going to think about it. By then, our technology may have progressed even beyond what we can do now. And a lot of it’s just going to be open source — which I fully support: the sharing of science, the sharing of data, the sharing of models. But it’s one of these things where we, as scientists, should take care that what we release is done responsibly.
How easy is it for someone to replicate what you did? What would they need?
I don’t want to sound very sensationalist about this, but it is fairly easy for someone to replicate what we did.
If you were to Google generative models, you could find a number of put-together one-liner generative models that people have released for free. And then, if you were to search for toxicity datasets, there’s a large number of open-source tox datasets. So if you just combine those two things, and then you know how to code and build machine learning models — all that requires really is an internet connection and a computer — then, you could easily replicate what we did. And not just for VX, but for pretty much whatever other open-source toxicity datasets exist.
Of course, it does require some expertise. If somebody were to put this together without knowing anything about chemistry, they would ultimately probably generate stuff that was not very useful. And there’s still the next step of having to get those molecules synthesized. Finding a potential drug or potential new toxic molecule is one thing; the next step of synthesis — actually creating a new molecule in the real world — would be another barrier.
Right, there’s still some big leaps between what the AI comes up with and turning that into a real-world threat. What are the gaps there?
The big gap to start with is that you really don’t know if these molecules are actually toxic or not. There’s going to be some amount of false positives. If we’re walking ourselves through what a bad agent would be thinking or doing, they would have to make a decision on which of these new molecules they would want to synthesize ultimately.
As far as synthesis routes, this could be a make it or break it. If you find something that looks like a chemical warfare agent and try to get that synthesized, chances are it’s not going to happen. A lot of the chemical building blocks of these chemical warfare agents are well known and are watched. They’re regulated. But there’s so many synthesis companies. As long as it doesn’t look like a chemical warfare agent, they’re most likely going to just synthesize it and send it right back because who knows what the molecule is being used for, right?
You get at this later in the paper, but what can be done to prevent this kind of misuse of AI? What safeguards would you like to see established?
For context, there are more and more policies about data sharing. And I completely agree with it because it opens up more avenues for research. It allows other researchers to see your data and use it for their own research. But at the same time, that also includes things like toxicity datasets and toxicity models. So it’s a little hard to figure out a good solution for this problem.
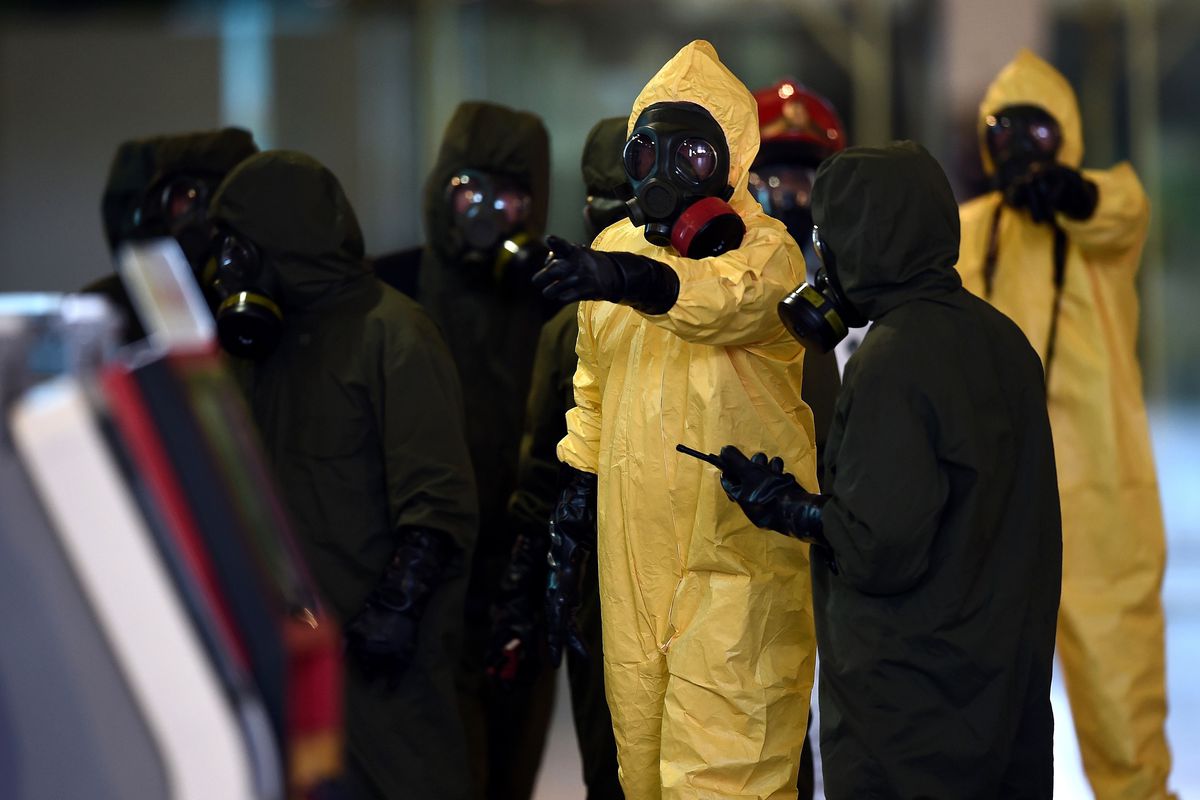
We looked over towards Silicon Valley: there’s a group called OpenAI; they released a top-of-the-line language model called GPT-3. It’s almost like a chatbot; it basically can generate sentences and text that is almost indistinguishable from humans. They actually let you use it for free whenever you want, but you have to get a special access token from them to do so. At any point, they could cut off your access from those models. We were thinking something like that could be a useful starting point for potentially sensitive models, such as toxicity models.
Science is all about open communication, open access, open data sharing. Restrictions are antithetical to that notion. But a step going forward could be to at least responsibly account for who’s using your resources.
Your paper also says that “[w]ithout being overly alarmist, this should serve as a wake-up call for our colleagues” — what is it that you want your colleagues to wake up to? And what do you think that being overly alarmist would look like?
We just want more researchers to acknowledge and be aware of potential misuse. When you start working in the chemistry space, you do get informed about misuse of chemistry, and you’re sort of responsible for making sure you avoid that as much as possible. In machine learning, there’s nothing of the sort. There’s no guidance on misuse of the technology.
So putting that awareness out there could help people really be mindful of the issue. Then it’s at least talked about in broader circles and can at least be something that we watch out for as we get better and better at building toxicity models.
I don’t want to propose that machine learning AI is going to start creating toxic molecules and there’s going to be a slew of new biochemical warfare agents just around the corner. That somebody clicks a button and then, you know, chemical warfare agents just sort of appear in their hand.
I don’t want to be alarmist in saying that there’s going to be AI-driven chemical warfare. I don’t think that’s the case now. I don’t think it’s going to be the case anytime soon. But it’s something that’s starting to become a possibility.
https://www.theverge.com/2022/3/17/22983197/ai-new-possible-chemical-weapons-generative-models-vx